Dealing With Missing Values Missing Values In A Data Science Project
Missing Data Values And How To Handle It Pdf Statistics Applied Before handling missing values, one should understand why and where data is missing. d.b.rubin describes three types of missing data based on the mechanism of missingness. Explore various techniques to efficiently handle missing values and their implementations in python.
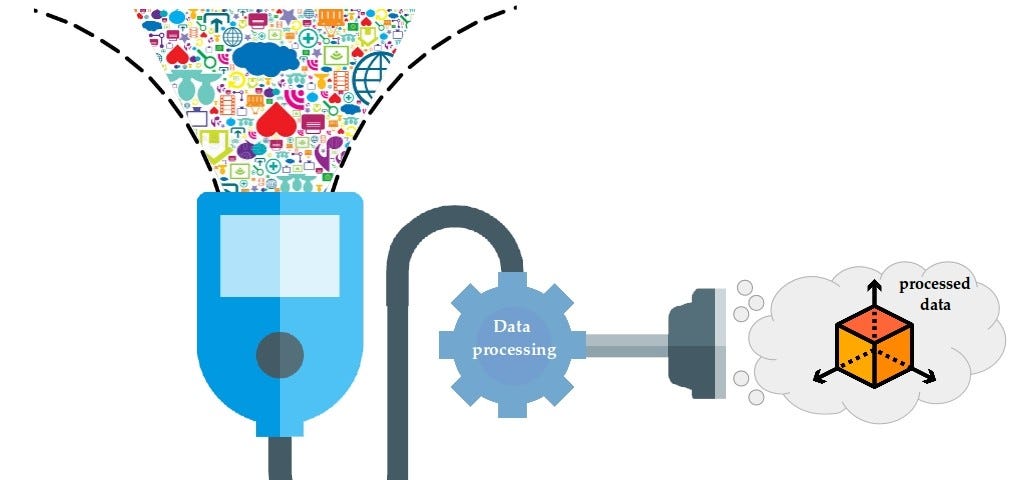
Handling Missing Values Perspectives On Data Science Medium Missing values are a common issue in machine learning. this occurs when a particular variable lacks data points, resulting in incomplete information and potentially harming the accuracy and dependability of your models. it is essential to address missing values efficiently to ensure strong and impartial results in your machine learning projects. Learn top techniques to handle missing values effectively in data science projects. from simple deletion to predictive imputation, master essential methods. In this article, i will discuss the common types of outliers and missing values one may encounter, explain why they are problematic for analysis and modeling, and provide step by step guidance.
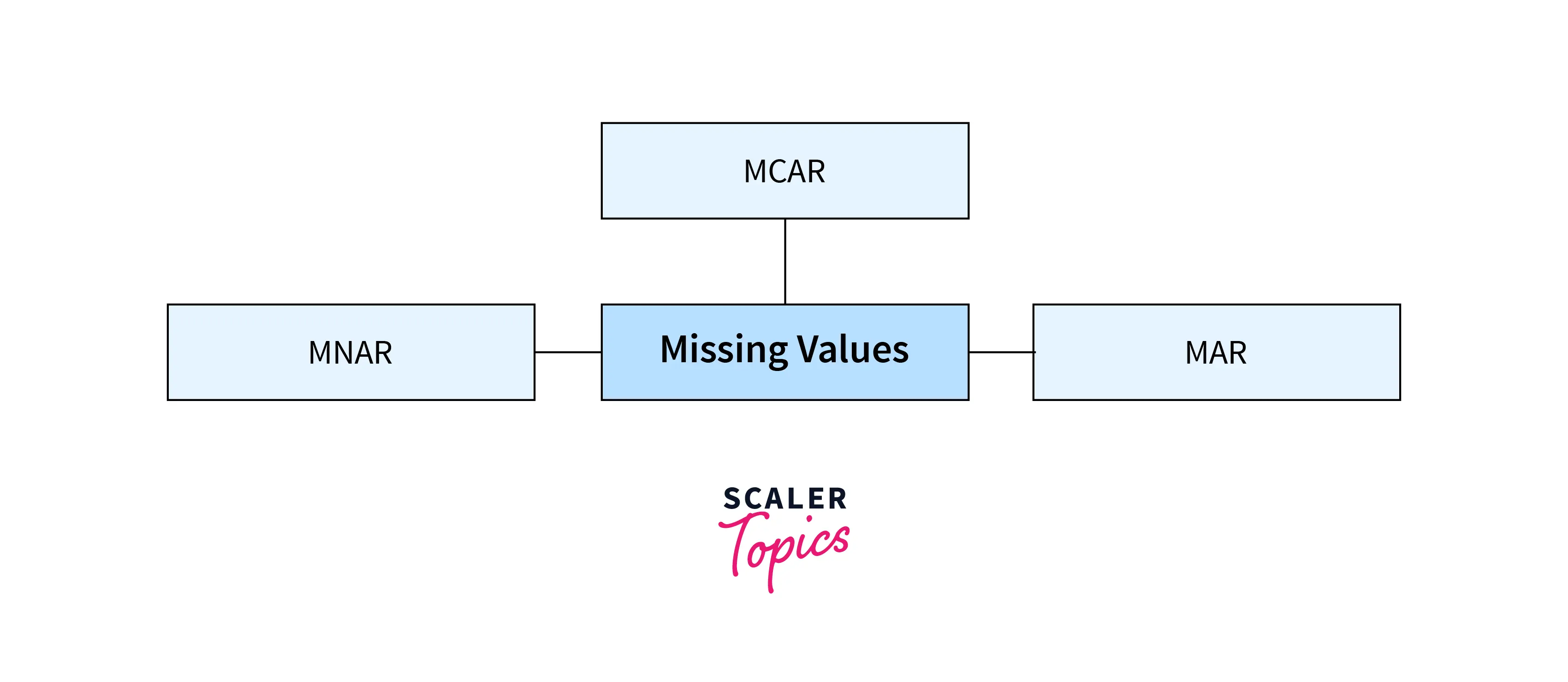
Handling Missing Values Categorical Numerical Scaler Topics In this article, i will discuss the common types of outliers and missing values one may encounter, explain why they are problematic for analysis and modeling, and provide step by step guidance.

Data Science Blog 365 Data Science
Comments are closed.