Eea Esem 2022 Victor Chernozhukov Mit Using Machine Learning For Causal Inference In Economics
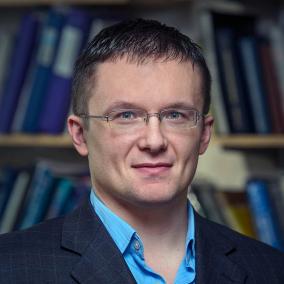
Victor Chernozhukov Mit Economics Victor chernozhukov works in econometrics and mathematical statistics, with much of recent work focusing on the quantification of uncertainty in very high di. Victor chernozhukov gives his session at eea esem using machine learning for causal inference in economics. slides available at bit.ly 3akd7yf.
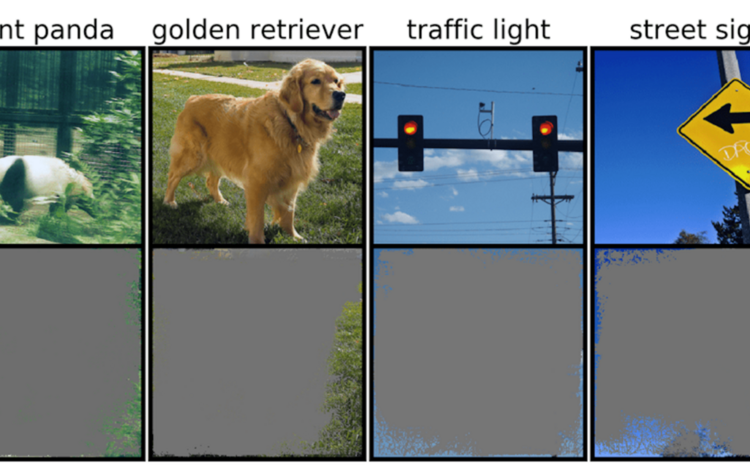
News Mit Eecs This paper provides estimation and inference methods for the best linear predictor (approximation) of a structural function, such as conditional average structural and treatment effects, and structural derivatives, based on modern machine learning tools. We provide an introduction to the use of machine learning methods in econometrics and how these methods can be employed to assist in causal inference. we begin with an extended presentation of the lasso (least absolute shrinkage and selection operator) of tibshirani. We focus on the double machine learning, causal forest, and generic machine learning methods, in the context of both average and heterogeneous treatment effects. we illustrate the implementation of these methods in a variety of settings and highlight the relevance and value added relative to traditional methods used in the original studies. Plugging machine learners into identifying equations can lead to poor inference due to bias from regularization and or model selection. this paper gives automatic debiasing for linear and nonlinear functions of regressions. the debiasing is automatic in using lasso and the function of interest without the full form of the bias correction.
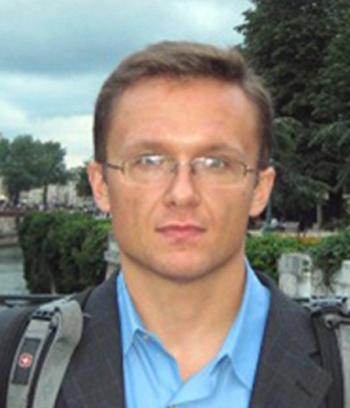
Victor Chernozhukov Idss We focus on the double machine learning, causal forest, and generic machine learning methods, in the context of both average and heterogeneous treatment effects. we illustrate the implementation of these methods in a variety of settings and highlight the relevance and value added relative to traditional methods used in the original studies. Plugging machine learners into identifying equations can lead to poor inference due to bias from regularization and or model selection. this paper gives automatic debiasing for linear and nonlinear functions of regressions. the debiasing is automatic in using lasso and the function of interest without the full form of the bias correction. Furthermore, we use debiased machine learning to provide flexible and efficient statistical inference on learnable components of the bounds. finally, empirical examples demonstrate the usefulness of the approach. Generic machine learning inference on heterogenous treatment effects in randomized experiments, arxiv, 2020. paper victor chernozhukov, mert demirer, esther duflo, iván fernández val. An introduction to the emerging fusion of machine learning and causal inference. the book introduces ideas from classical structural equation models (sems) and their modern ai equivalent, directed acyclical graphs (dags) and structural causal models (scms), and presents debiased machine learning methods to do inference in such models using. Therefore, simple plausibility judgments on the maximum explanatory power of omitted variables (in explaining treatment and outcome variation) are sufficient to place overall bounds on the size of the bias. furthermore, we use debiased machine learning to provide flexible and efficient statistical inference on learnable components of the bounds.
Github Andrzejczukm Machine Learning This Repository Contains Furthermore, we use debiased machine learning to provide flexible and efficient statistical inference on learnable components of the bounds. finally, empirical examples demonstrate the usefulness of the approach. Generic machine learning inference on heterogenous treatment effects in randomized experiments, arxiv, 2020. paper victor chernozhukov, mert demirer, esther duflo, iván fernández val. An introduction to the emerging fusion of machine learning and causal inference. the book introduces ideas from classical structural equation models (sems) and their modern ai equivalent, directed acyclical graphs (dags) and structural causal models (scms), and presents debiased machine learning methods to do inference in such models using. Therefore, simple plausibility judgments on the maximum explanatory power of omitted variables (in explaining treatment and outcome variation) are sufficient to place overall bounds on the size of the bias. furthermore, we use debiased machine learning to provide flexible and efficient statistical inference on learnable components of the bounds.
Github Gene1974 Machinelearning 清华大学电子工程系研究生课程 机器学习 An introduction to the emerging fusion of machine learning and causal inference. the book introduces ideas from classical structural equation models (sems) and their modern ai equivalent, directed acyclical graphs (dags) and structural causal models (scms), and presents debiased machine learning methods to do inference in such models using. Therefore, simple plausibility judgments on the maximum explanatory power of omitted variables (in explaining treatment and outcome variation) are sufficient to place overall bounds on the size of the bias. furthermore, we use debiased machine learning to provide flexible and efficient statistical inference on learnable components of the bounds.
Comments are closed.