Figure 1 From Autonomous Multi Hop Network Using Pso Based Mobile Robot
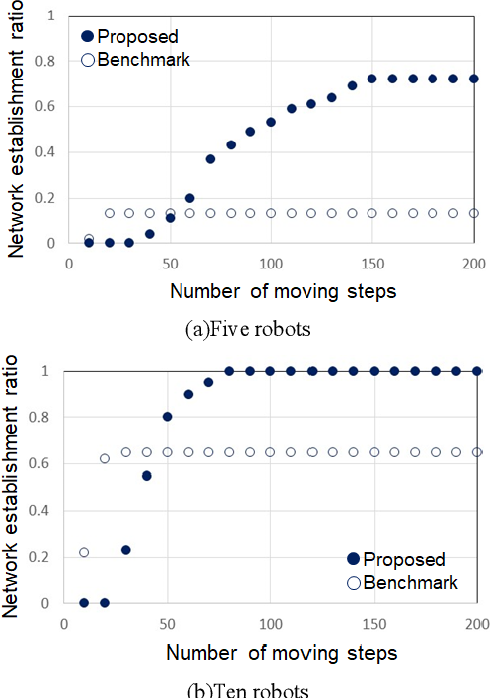
Figure 2 From Autonomous Multi Hop Network Using Pso Based Mobile Robot In this study, a mobile robot swarm algorithm based on particle swarm optimization is proposed, where the unique moving target technique is introduced to efficiently establish an autonomous multi hop network between a target position where an event occurs and an operator at a remote base station, e.g., for monitoring real time information and. It is demonstrated that a mobile robot swarm using the proposed algorithm can establish an efficient multi hop network with a higher success rate than the existing algorithm and can establish a flexible multi hop network that adjusts to a placement pattern of obstacles without collisions.
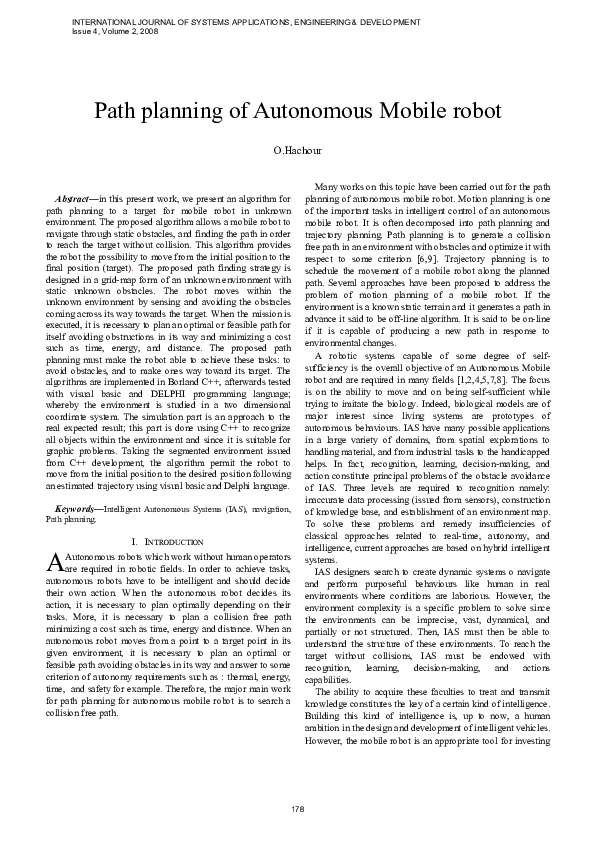
Pdf Path Planning Of Autonomous Mobile Robot Path planning is an important process for autonomous mobile robots, as this helps robots move from a starting point to a destination without hitting any obstacles. generally, path planning for autonomous mobile robots is divided into two categories: global path planning and local path planning. Inspired by such requirements, this article proposes a moving distance minimized pso (mpso) for a mobile robot swarm to minimize the total moving distance of its robots while performing optimization. Generate trajectories for an autonomous mobile robot when it senses obstacles in its environment. the present research work develops a pso based system architecture for obtaining optimal path trajectories when the robot senses obstacles within its work space. In this paper, the selection of target points for mobile robots is transformed into solving tsp problem, and the improved particle swarm optimization algorithm is used to solve tsp problem and the optimization of path planning objective function.
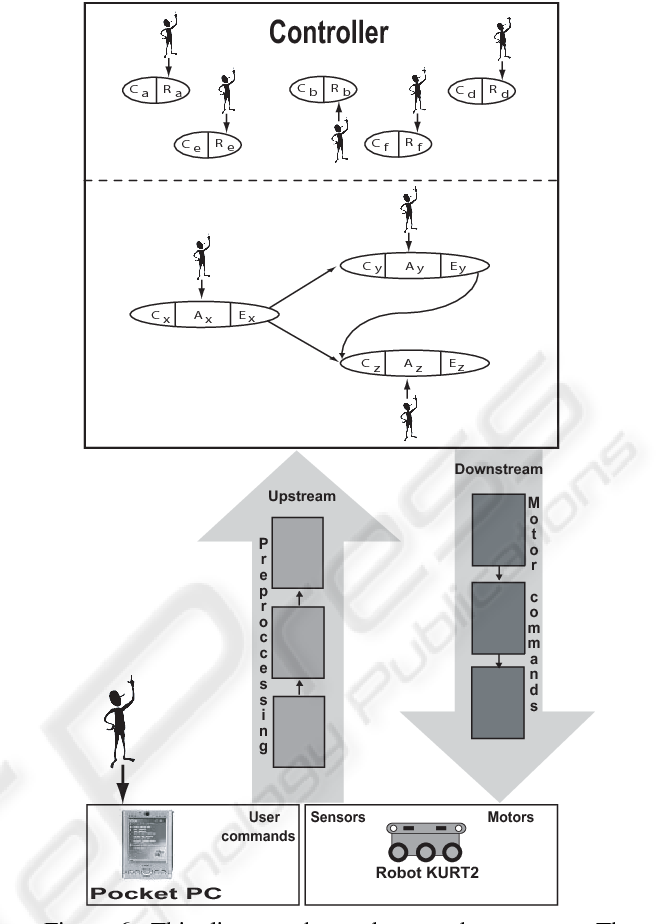
Figure 1 From An Implementation On An Autonomous Mobile Robot Generate trajectories for an autonomous mobile robot when it senses obstacles in its environment. the present research work develops a pso based system architecture for obtaining optimal path trajectories when the robot senses obstacles within its work space. In this paper, the selection of target points for mobile robots is transformed into solving tsp problem, and the improved particle swarm optimization algorithm is used to solve tsp problem and the optimization of path planning objective function. The main aim of this paper is to solve a path planning problem for an autonomous mobile robot in static and dynamic environments. the problem is solved by determining the collision free path that satisfies the chosen criteria for shortest distance and path smoothness. This paper presents an enforcing multi hop network connectivity algorithm experimentally validated using a modified version of the darwinian particle swarm optimization (dpso), denoted as rdpso (robotic dpso) on groups of simulated robots performing a distributed exploration task. In brief, compared with existing methods, the robot can reasonably plan a shorter and smoother path with the aid of pso based apf, meanwhile quickly react to the moving obstacles and avoid them by fuzzy based dwa. finally, a static multi obstacle environment and two dynamic scenarios with moving obstacles are simulated to verify the. To overcome the limitations of particle swarm optimization (pso) in mobile robot path planning, including issues such as premature convergence and sensitivity to local optima, this study proposes a novel approach, dynamic multipopulation particle swarm optimization (dmpso).
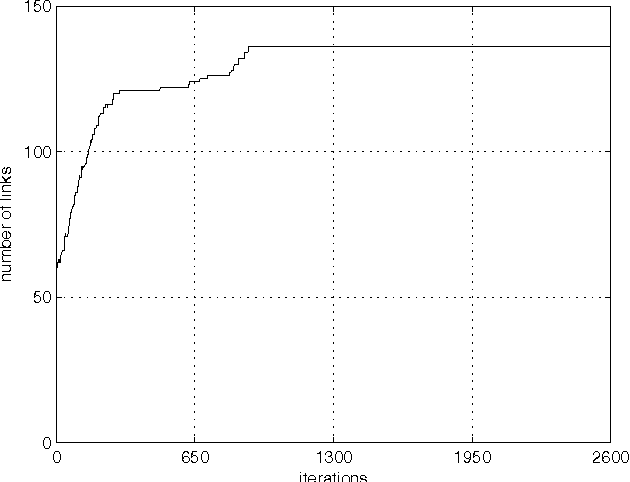
Figure 2 From Navigation Of Autonomous Mobile Robots In Connected The main aim of this paper is to solve a path planning problem for an autonomous mobile robot in static and dynamic environments. the problem is solved by determining the collision free path that satisfies the chosen criteria for shortest distance and path smoothness. This paper presents an enforcing multi hop network connectivity algorithm experimentally validated using a modified version of the darwinian particle swarm optimization (dpso), denoted as rdpso (robotic dpso) on groups of simulated robots performing a distributed exploration task. In brief, compared with existing methods, the robot can reasonably plan a shorter and smoother path with the aid of pso based apf, meanwhile quickly react to the moving obstacles and avoid them by fuzzy based dwa. finally, a static multi obstacle environment and two dynamic scenarios with moving obstacles are simulated to verify the. To overcome the limitations of particle swarm optimization (pso) in mobile robot path planning, including issues such as premature convergence and sensitivity to local optima, this study proposes a novel approach, dynamic multipopulation particle swarm optimization (dmpso).
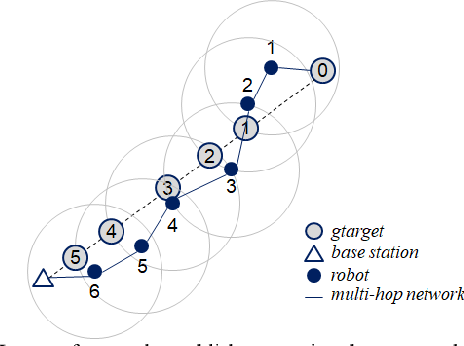
Figure 1 From Autonomous Multi Hop Network Using Pso Based Mobile Robot In brief, compared with existing methods, the robot can reasonably plan a shorter and smoother path with the aid of pso based apf, meanwhile quickly react to the moving obstacles and avoid them by fuzzy based dwa. finally, a static multi obstacle environment and two dynamic scenarios with moving obstacles are simulated to verify the. To overcome the limitations of particle swarm optimization (pso) in mobile robot path planning, including issues such as premature convergence and sensitivity to local optima, this study proposes a novel approach, dynamic multipopulation particle swarm optimization (dmpso).
Comments are closed.