Figure 5 From Pan Cancer Integrative Histology Genomic Analysis Via
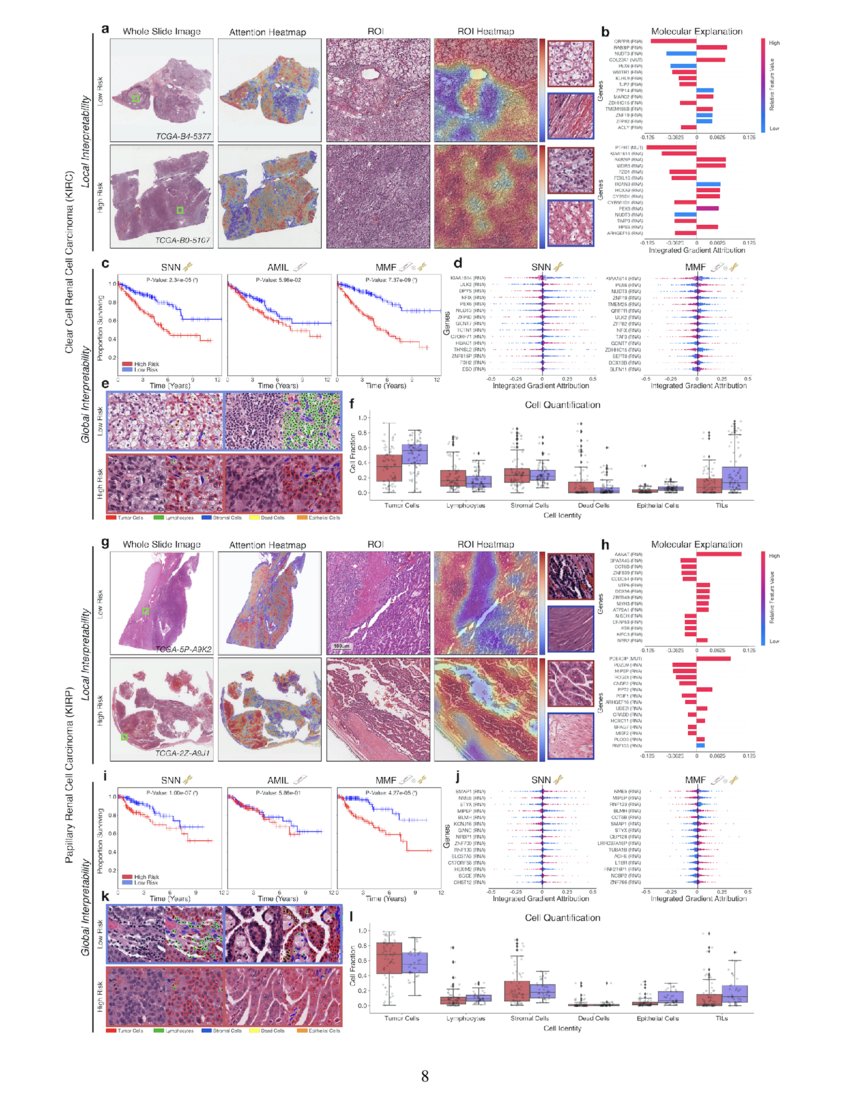
Pan Cancer Integrative Histology Genomic Analysis Via Interpretable We use multimodal deep learning to jointly examine pathology whole slide images and molecular profile data from 14 cancer types. our weakly supervised, multimodal deep learning algorithm is able to fuse these heterogeneous modalities to predict outcomes and discover prognostic features that correlate with poor and favorable outcomes. We present all analyses for morphological and molecular correlates of patient prognosis across the 14 cancer types at both a disease and a patient level in an interactive open access database to allow for further exploration, biomarker discovery, and feature assessment.
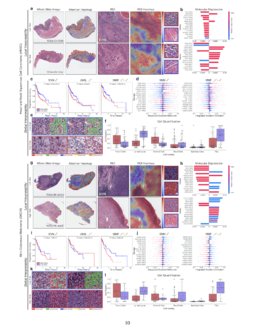
Pan Cancer Integrative Histology Genomic Analysis Via Interpretable We introduce a novel deep learning driven approach, catfusion, for pan cancer survival prediction by integrating multimodal histology genomic data. our methodology introduces several innovative elements. Quantitative performance, local model explanation, and global interpretability analyses of porpoise on lower grade gliomas (lggs) "pan cancer integrative histology genomic analysis via multimodal deep learning.". We used multimodal deep learning to integrate gigapixel whole slide pathology images, rna seq abundance, copy number variation, and mutation data from 5,720 patients across 14 major cancer types. Figure 5 shows that the integrated survclust solution outperformed individual platforms based on the cross validated logrank statistics for multiple cancer types including cervical cancer, head and neck cancer, papillary kidney cancer, lower grade glioma, and liver and endometrial cancers. in general, the integrated solutions always emerge at.
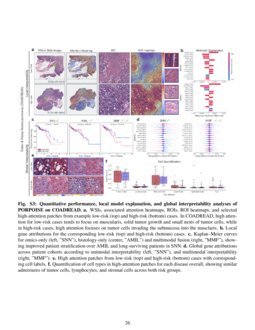
Pan Cancer Integrative Histology Genomic Analysis Via Interpretable We used multimodal deep learning to integrate gigapixel whole slide pathology images, rna seq abundance, copy number variation, and mutation data from 5,720 patients across 14 major cancer types. Figure 5 shows that the integrated survclust solution outperformed individual platforms based on the cross validated logrank statistics for multiple cancer types including cervical cancer, head and neck cancer, papillary kidney cancer, lower grade glioma, and liver and endometrial cancers. in general, the integrated solutions always emerge at. We present all analyses for morphological and molecular correlates of patient prognosis across the 14 cancer types at both a disease and a patient level in an interactive open access database to allow for further exploration, biomarker discovery, and feature assessment. The rapidly emerging field of deep learning based computational pathology has demonstrated promise in developing objective prognostic models from histology whole slide images. several studies have focused on predicting genomic and transcriptomic alterations from histology images. Chen et al. present a pan cancer analysis that uses deep learning to integrate whole slide pathology images and molecular features to predict cancer prognosis, with multimodal interpretability used to elucidate morphologic and molecular correlates of prognosis. This study explored the expression, prognostic value, and tumor infiltrating immune of mki67 in the tcga database by pan cancer, and then performed immunohistochemical, correlation analysis and prognostic analysis using 10028 patients of the top 10 cancer patients in china we collected.

Pan Cancer Integrative Histology Genomic Analysis Via Multimodal Deep We present all analyses for morphological and molecular correlates of patient prognosis across the 14 cancer types at both a disease and a patient level in an interactive open access database to allow for further exploration, biomarker discovery, and feature assessment. The rapidly emerging field of deep learning based computational pathology has demonstrated promise in developing objective prognostic models from histology whole slide images. several studies have focused on predicting genomic and transcriptomic alterations from histology images. Chen et al. present a pan cancer analysis that uses deep learning to integrate whole slide pathology images and molecular features to predict cancer prognosis, with multimodal interpretability used to elucidate morphologic and molecular correlates of prognosis. This study explored the expression, prognostic value, and tumor infiltrating immune of mki67 in the tcga database by pan cancer, and then performed immunohistochemical, correlation analysis and prognostic analysis using 10028 patients of the top 10 cancer patients in china we collected.

Pan Cancer Integrative Histology Genomic Analysis Via Multimodal Deep Chen et al. present a pan cancer analysis that uses deep learning to integrate whole slide pathology images and molecular features to predict cancer prognosis, with multimodal interpretability used to elucidate morphologic and molecular correlates of prognosis. This study explored the expression, prognostic value, and tumor infiltrating immune of mki67 in the tcga database by pan cancer, and then performed immunohistochemical, correlation analysis and prognostic analysis using 10028 patients of the top 10 cancer patients in china we collected.
Comments are closed.