Github Pilshan Awesome Continual Semantic Segmentation
Github Pilshan Awesome Continual Semantic Segmentation Contribute to pilshan awesome continual semantic segmentation development by creating an account on github. Extensive experimental evaluation on multiple autonomous driving datasets shows how the proposed method outperforms existing approaches, which prove to be ill equipped to deal with continual semantic segmentation under both task and domain shift.
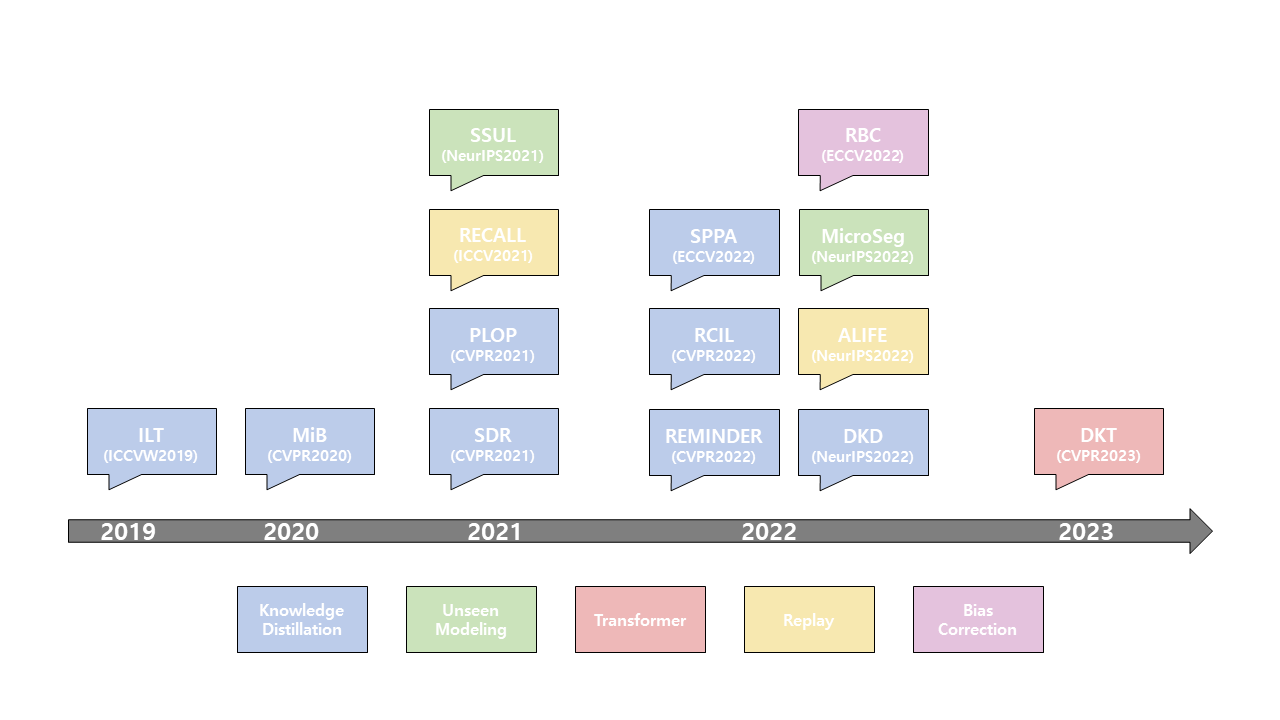
Github Pilshan Awesome Continual Semantic Segmentation Continual semantic segmentation (css), of which the dense prediction peculiarity makes it a challenging, intricate and burgeoning task. in this paper, we present a review of css, committing to building a comprehensive survey on problem formulations, primary challenges, universal datasets, neoteric theories and multifarious applications. Continual semantic segmentation (css) extends static semantic segmentation by incrementally introducing new classes for training. to alleviate the catastrophic forgetting issue in css, a memory buffer that stores a small number of samples from the previous classes is constructed for replay. Semantic segmentation plays a crucial role in enabling comprehensive scene understanding for robotic systems. however, generating annotations is challenging, requiring labels for every pixel in an image. To extend the applicability of semantic segmentation methods, we introduce a novel, scalable segmentation architecture called scaleseg, designed to adapt the incremental scenarios. the architecture of scaleseg consists of a series of prototypes updated by online contrastive clustering.
Github Teakinboyewa Semantic Segmentation Semantic segmentation plays a crucial role in enabling comprehensive scene understanding for robotic systems. however, generating annotations is challenging, requiring labels for every pixel in an image. To extend the applicability of semantic segmentation methods, we introduce a novel, scalable segmentation architecture called scaleseg, designed to adapt the incremental scenarios. the architecture of scaleseg consists of a series of prototypes updated by online contrastive clustering. A survey on continual semantic segmentation: theory, challenge, method and application. bo yuan 1,2, danpei zhao 1,2 1 airvic lab, beihang university 2 tianmushan laboratory, hangzhou |. Recall: replay based continual learning in semantic segmentation (iccv, 2021) few shot and continual learning with attentive independent mechanisms (iccv, 2021) learning with selective forgetting (ijcai, 2021) continuous coordination as a realistic scenario for lifelong learning (icml, 2021). Contribute to pilshan awesome continual semantic segmentation development by creating an account on github. 本文对现有的css方法进行了调研、分类和比较,并在相关数据集上的定性和定量对比。 根据持续语义分割的场景,我们还把css任务分为了四种,分别是任务增量(task incremental css)、域增量(domain incremental css)、类别增量(class incremental css)和模态增量(modality incremental css)。 这四种任务涵盖了持续语义分割多样化的应用场景和发展趋势。 此外,本文还建立了一个css基准,其中包括代表性文献、评估结果和复制实验,仓库已经开源。 如下图所示,根据是否需要存储旧数据,css可以分为基于回放的方法(data replay)和不依赖旧数据的方法(data free)两类。.
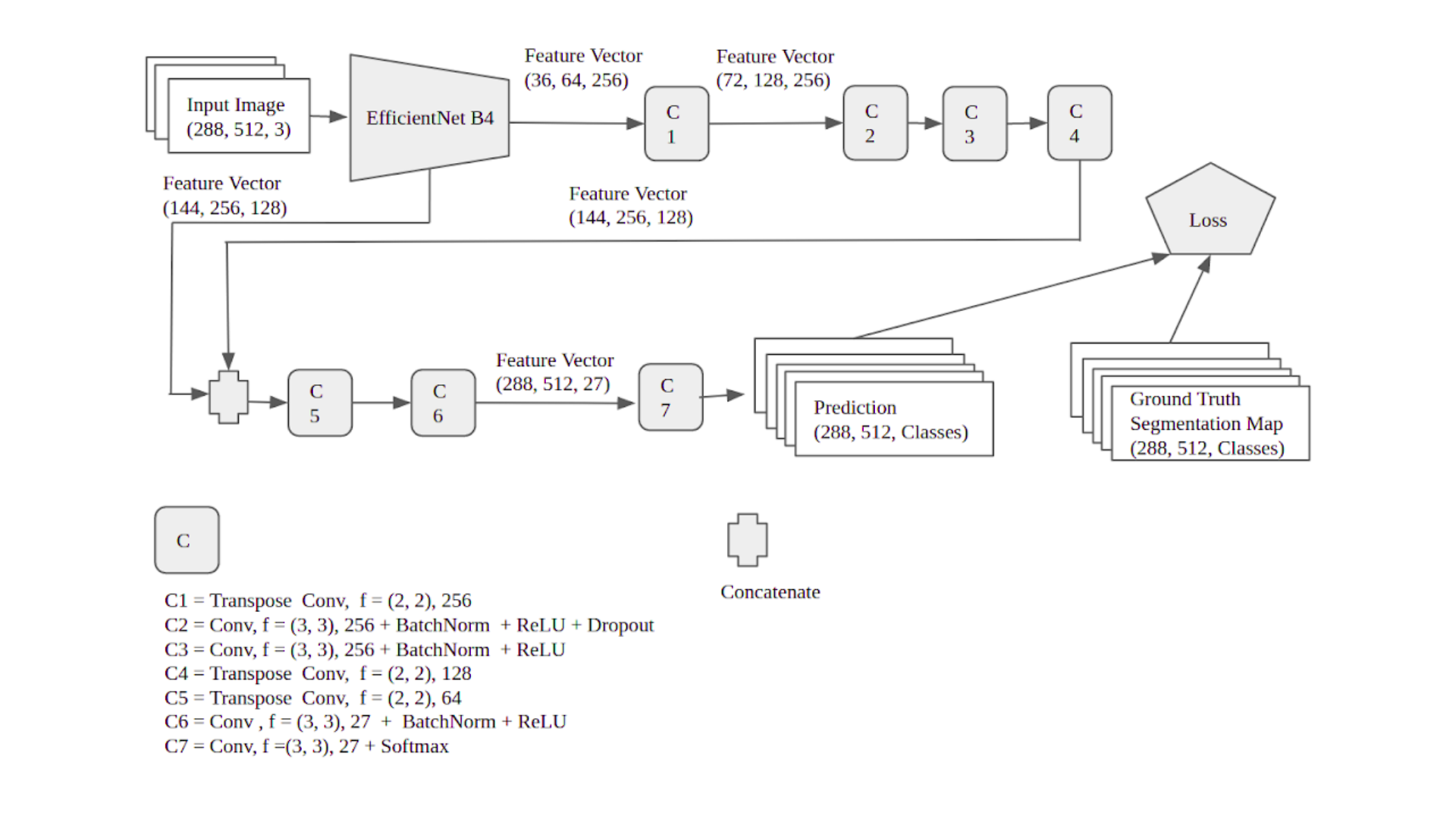
Github Himgautam Semantic Segmentation A survey on continual semantic segmentation: theory, challenge, method and application. bo yuan 1,2, danpei zhao 1,2 1 airvic lab, beihang university 2 tianmushan laboratory, hangzhou |. Recall: replay based continual learning in semantic segmentation (iccv, 2021) few shot and continual learning with attentive independent mechanisms (iccv, 2021) learning with selective forgetting (ijcai, 2021) continuous coordination as a realistic scenario for lifelong learning (icml, 2021). Contribute to pilshan awesome continual semantic segmentation development by creating an account on github. 本文对现有的css方法进行了调研、分类和比较,并在相关数据集上的定性和定量对比。 根据持续语义分割的场景,我们还把css任务分为了四种,分别是任务增量(task incremental css)、域增量(domain incremental css)、类别增量(class incremental css)和模态增量(modality incremental css)。 这四种任务涵盖了持续语义分割多样化的应用场景和发展趋势。 此外,本文还建立了一个css基准,其中包括代表性文献、评估结果和复制实验,仓库已经开源。 如下图所示,根据是否需要存储旧数据,css可以分为基于回放的方法(data replay)和不依赖旧数据的方法(data free)两类。.
Comments are closed.