Machine Learning Helps Researchers Develop Perovskite Solar Cells With
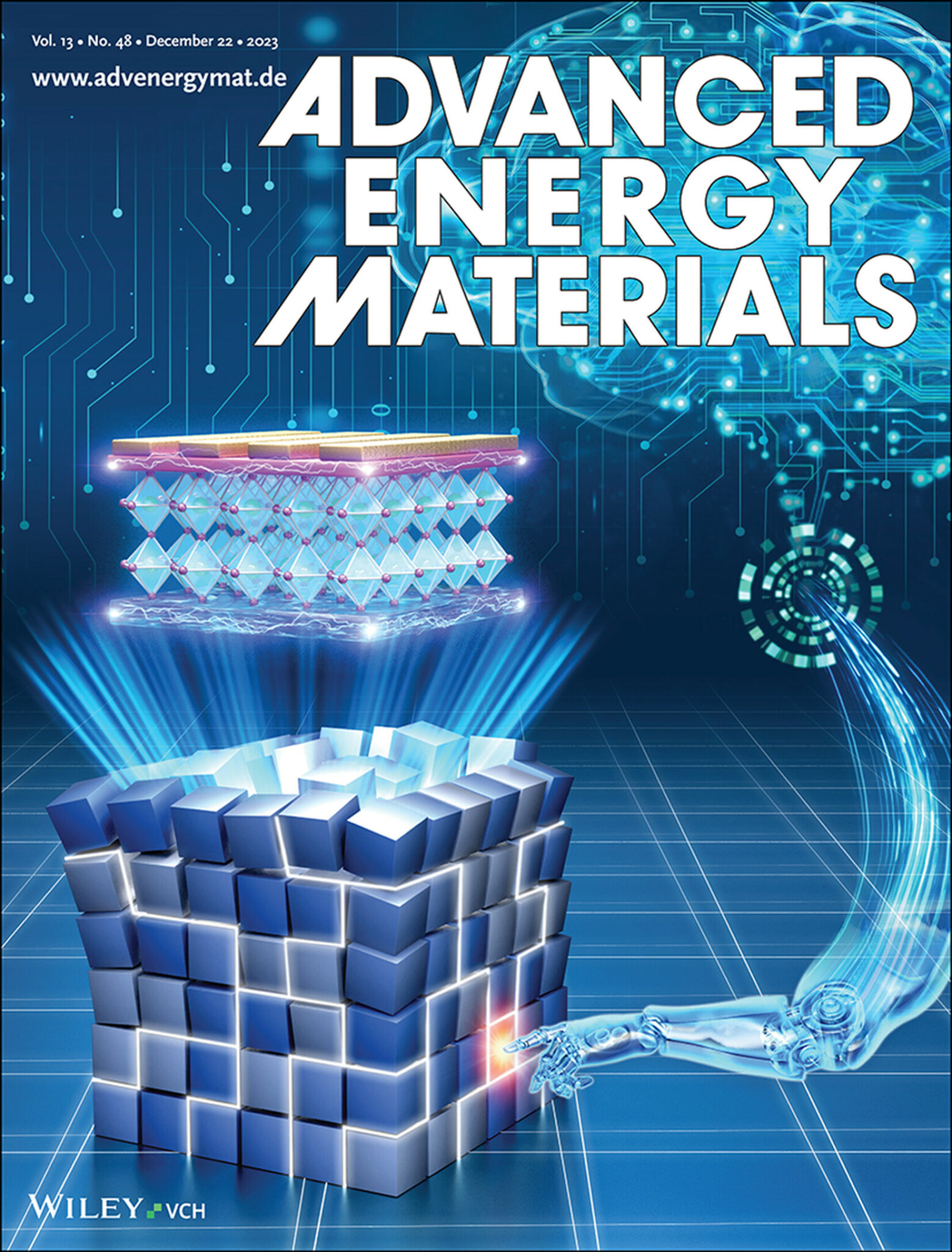
Optimizing Perovskite Thin Film Parameter Spaces With Machine Learning Machine learning (ml) is emerging to accelerate the exploration and development of new perovskite solar cells (pscs). herein, we use the ml with advanced algorithms to predict five unexplored (fapbi3) x (mapbbr 2.8 cl 0.2) 1 x perovskites with low bandgaps for experimental guidance. An international team of scientists has used machine learning to help them develop perovskite solar cells with near record efficiency. in their paper published in the journal science, the group describes how they used the machine learning algorithm to help them find new hole transporting materials to improve the efficiency of perovskite solar.
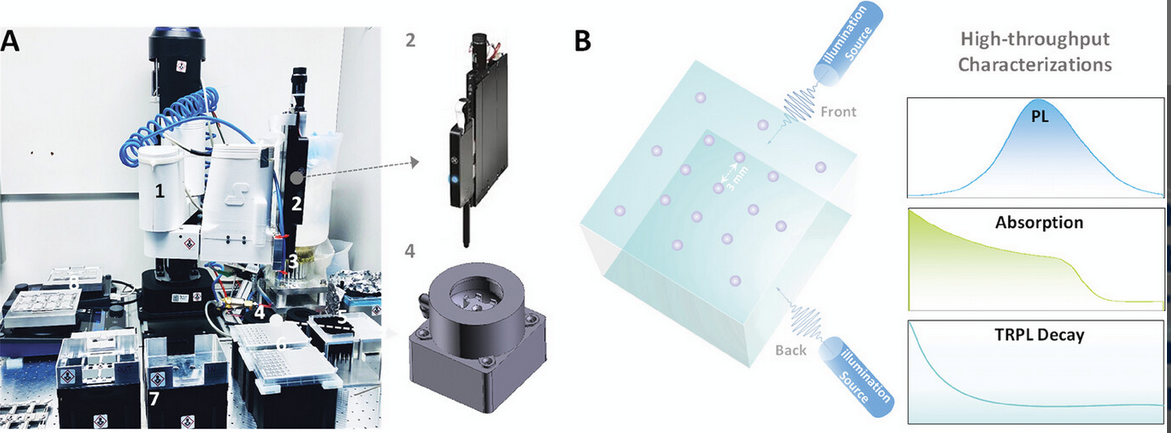
Optimizing Perovskite Thin Film Parameter Spaces With Machine Learning To aid future research in using ml to guide perovskite solar cell design and manufacturing, we have created an open source data cleaning and ml pipeline leveraging the perovskite database project (pdp). the pdp offers a large open source repository of psc device information with detailed documentation. Over the last few years there has been an increasing number of papers using machine learning (ml) as a tool to aid research directed towards perovskite solar cells. this review provides an overview of this recent development with a focus on the type of questions and narratives being explored, which type of data that may be useful, and how to. The research process of perovskite solar cells combined with machine learning includes four main parts: data collection, model training, predicting target attributes, and experimental verification. Zhu et al. combine machine learning with dft computational data to efficiently predict properties across a large dataset of perovskite solar cells, facilitating the identification of the most stable and efficient perovskite materials.
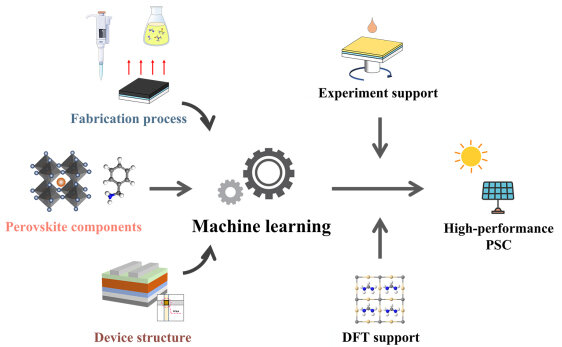
Predicting The Device Performance Of Perovskite Solar Cells Through The research process of perovskite solar cells combined with machine learning includes four main parts: data collection, model training, predicting target attributes, and experimental verification. Zhu et al. combine machine learning with dft computational data to efficiently predict properties across a large dataset of perovskite solar cells, facilitating the identification of the most stable and efficient perovskite materials. A hole transporting layer for perovskite solar cells with near record efficiency has been developed using a machine learning algorithm. the work explored a vast region of chemical. This review paper explores how machine learning (ml) approaches are improving the different aspects of perovskite solar cells (pscs) research. it presents compelling case studies about material inves. Researchers in australia have harnessed ai to produce solar cells from the mineral perovskite in just a matter of weeks, bypassing years of human labour and human error to optimise the cells. Usage of machine learning (ml) into psc research is significantly accelerated their holistic understanding of device requisite properties. ml techniques are increasingly employed to discover stable perovskite materials, optimize device architecture and processing, and analyze psc characterization data.

Data Of Perovskite Solar Cells Used For Machine Learning Training A hole transporting layer for perovskite solar cells with near record efficiency has been developed using a machine learning algorithm. the work explored a vast region of chemical. This review paper explores how machine learning (ml) approaches are improving the different aspects of perovskite solar cells (pscs) research. it presents compelling case studies about material inves. Researchers in australia have harnessed ai to produce solar cells from the mineral perovskite in just a matter of weeks, bypassing years of human labour and human error to optimise the cells. Usage of machine learning (ml) into psc research is significantly accelerated their holistic understanding of device requisite properties. ml techniques are increasingly employed to discover stable perovskite materials, optimize device architecture and processing, and analyze psc characterization data.

Machine Learning Helps Researchers Develop Perovskite Solar Cells With Researchers in australia have harnessed ai to produce solar cells from the mineral perovskite in just a matter of weeks, bypassing years of human labour and human error to optimise the cells. Usage of machine learning (ml) into psc research is significantly accelerated their holistic understanding of device requisite properties. ml techniques are increasingly employed to discover stable perovskite materials, optimize device architecture and processing, and analyze psc characterization data.

Machine Learning Assisted Fabrication Of Pcbm Perovskite Solar Cells
Comments are closed.