Pan Cancer Integrative Histology Genomic Analysis Via Interpretable
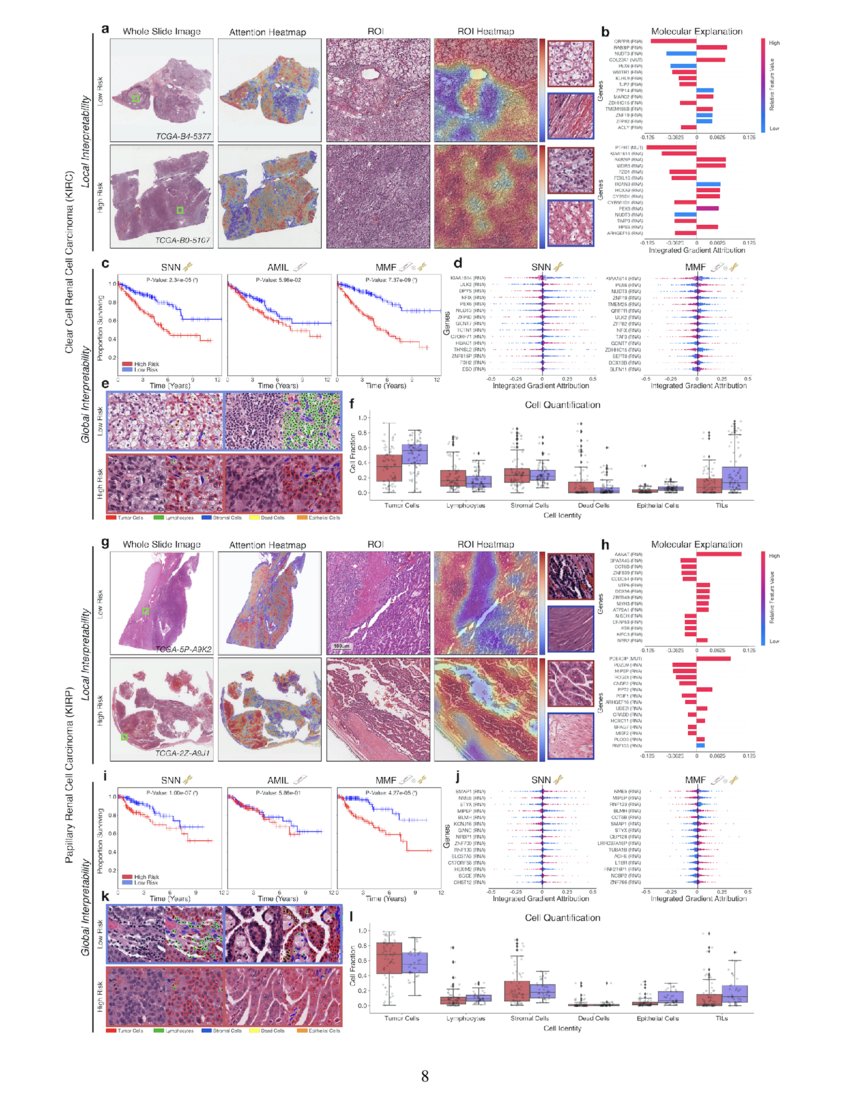
Pan Cancer Integrative Histology Genomic Analysis Via Interpretable In this study, we present a method for interpretable, weakly supervised, multimodal deep learning that integrates wsis and molecular profile data for cancer prognosis, which we trained and validated on 6,592 wsis from 5,720 patients with paired molecular profile data across 14 cancer types, and compared our method with unimodal deep learning. We use multimodal deep learning to jointly examine pathology whole slide images and molecular profile data from 14 cancer types. our weakly supervised, multimodal deep learning algorithm is able to fuse these heterogeneous modalities to predict outcomes and discover prognostic features that correlate with poor and favorable outcomes.
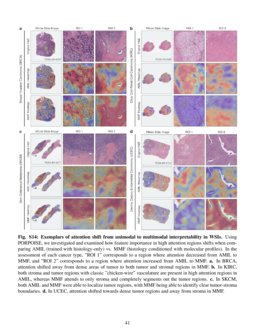
Pan Cancer Integrative Histology Genomic Analysis Via Interpretable We used multimodal deep learning to integrate gigapixel whole slide pathology images, rna seq abundance, copy number variation, and mutation data from 5,720 patients across 14 major cancer types. our interpretable, weakly supervised, multimodal deep learning algorithm is able to fuse these heterogeneous modalities for predicting outcomes and. Tl;dr we present an interpretable, weakly supervised, multimodal deep learning algorithm that integrates whole slide images (wsis) and molecular profile features for cancer prognosis. we validate our method on 14 cancer types, and extract both local and global patterns of morphological and molecular feature importances in each cancer type. Chen et al. present a pan cancer analysis that uses deep learning to integrate whole slide pathology images and molecular features to predict cancer prognosis, with multimodal interpretability used to elucidate morphologic and molecular correlates of prognosis. chen et al., 2022, cancer cell 40, 865–878 august 8, 2022 ª 2022 the author(s). The rapidly emerging field of deep learning based computational pathology has demonstrated promise in developing objective prognostic models from histology whole slide images. several studies have focused on predicting genomic and transcriptomic alterations from histology images.
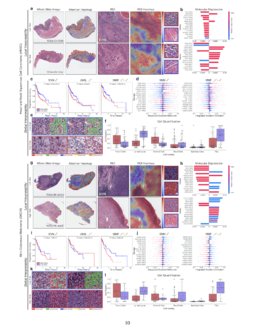
Pan Cancer Integrative Histology Genomic Analysis Via Interpretable Chen et al. present a pan cancer analysis that uses deep learning to integrate whole slide pathology images and molecular features to predict cancer prognosis, with multimodal interpretability used to elucidate morphologic and molecular correlates of prognosis. chen et al., 2022, cancer cell 40, 865–878 august 8, 2022 ª 2022 the author(s). The rapidly emerging field of deep learning based computational pathology has demonstrated promise in developing objective prognostic models from histology whole slide images. several studies have focused on predicting genomic and transcriptomic alterations from histology images. Chen et al. present a pan cancer analysis that uses deep learning to integrate whole slide pathology images and molecular features to predict cancer prognosis, with multimodal interpretability used to elucidate morphologic and molecular correlates of prognosis. Pan cancer integrative histology genomic analysis via interpretable multimodal deep learning richard j. chen , ming y. lu, drew f. k. williamson, tiffany y. chen, , jana lipkova, muhammad shaban, maha. We used multimodal deep learning to integrate gigapixel whole slide pathology images, rna seq abundance, copy number variation, and mutation data from 5,720 patients across 14 major cancer types. We used multimodal deep learning to integrate gigapixel whole slide pathology images, rna seq abundance, copy number variation, and mutation data from 5,720 patients across 14 major cancer types.
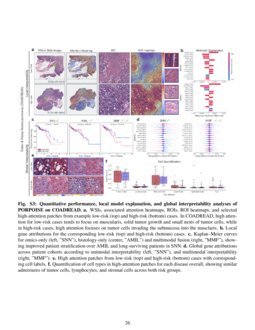
Pan Cancer Integrative Histology Genomic Analysis Via Interpretable Chen et al. present a pan cancer analysis that uses deep learning to integrate whole slide pathology images and molecular features to predict cancer prognosis, with multimodal interpretability used to elucidate morphologic and molecular correlates of prognosis. Pan cancer integrative histology genomic analysis via interpretable multimodal deep learning richard j. chen , ming y. lu, drew f. k. williamson, tiffany y. chen, , jana lipkova, muhammad shaban, maha. We used multimodal deep learning to integrate gigapixel whole slide pathology images, rna seq abundance, copy number variation, and mutation data from 5,720 patients across 14 major cancer types. We used multimodal deep learning to integrate gigapixel whole slide pathology images, rna seq abundance, copy number variation, and mutation data from 5,720 patients across 14 major cancer types.
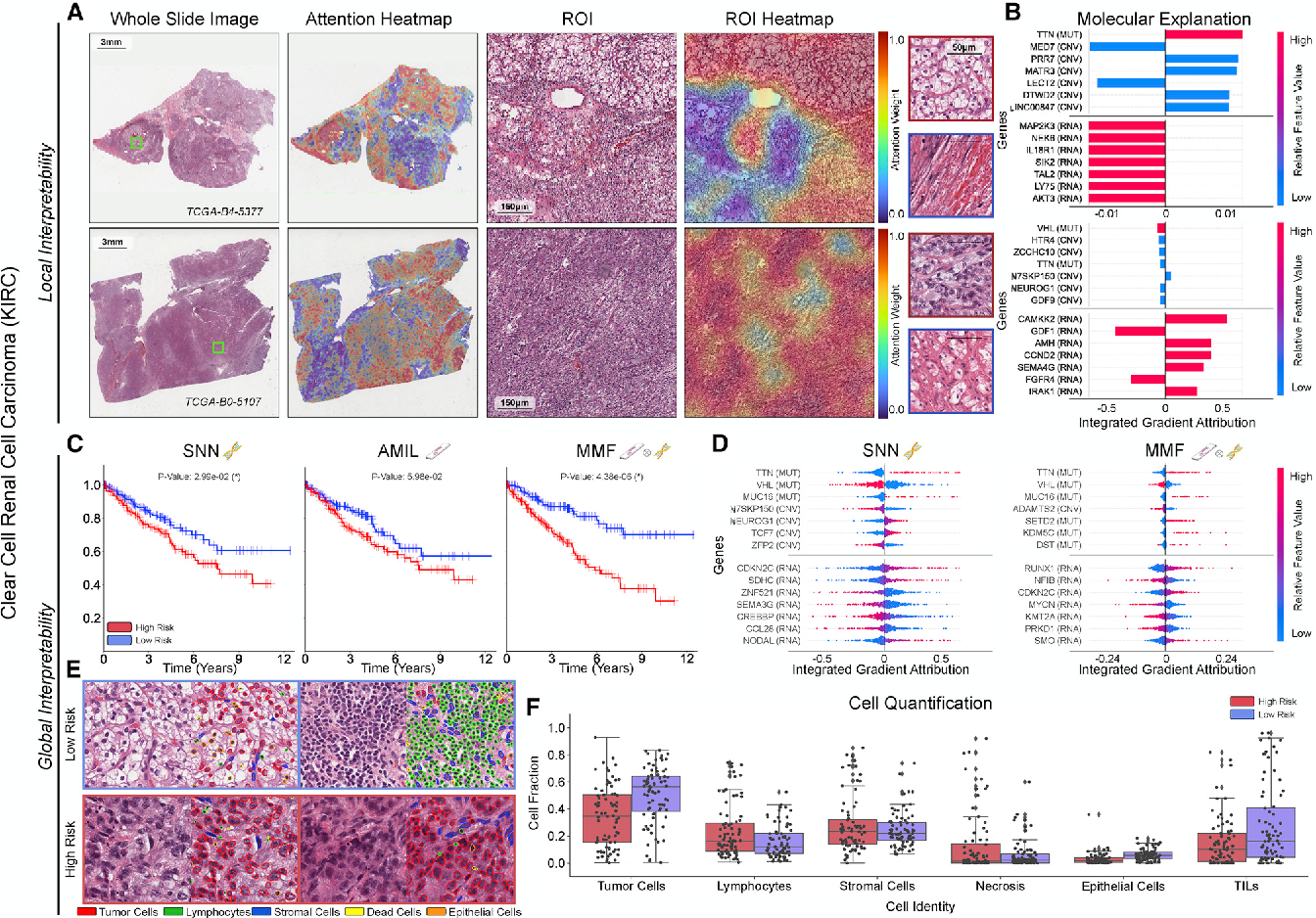
Figure 3 From Pan Cancer Integrative Histology Genomic Analysis Via We used multimodal deep learning to integrate gigapixel whole slide pathology images, rna seq abundance, copy number variation, and mutation data from 5,720 patients across 14 major cancer types. We used multimodal deep learning to integrate gigapixel whole slide pathology images, rna seq abundance, copy number variation, and mutation data from 5,720 patients across 14 major cancer types.
Comments are closed.