Paper Review Pan Cancer Integrative Histology Genomic Analysis Via
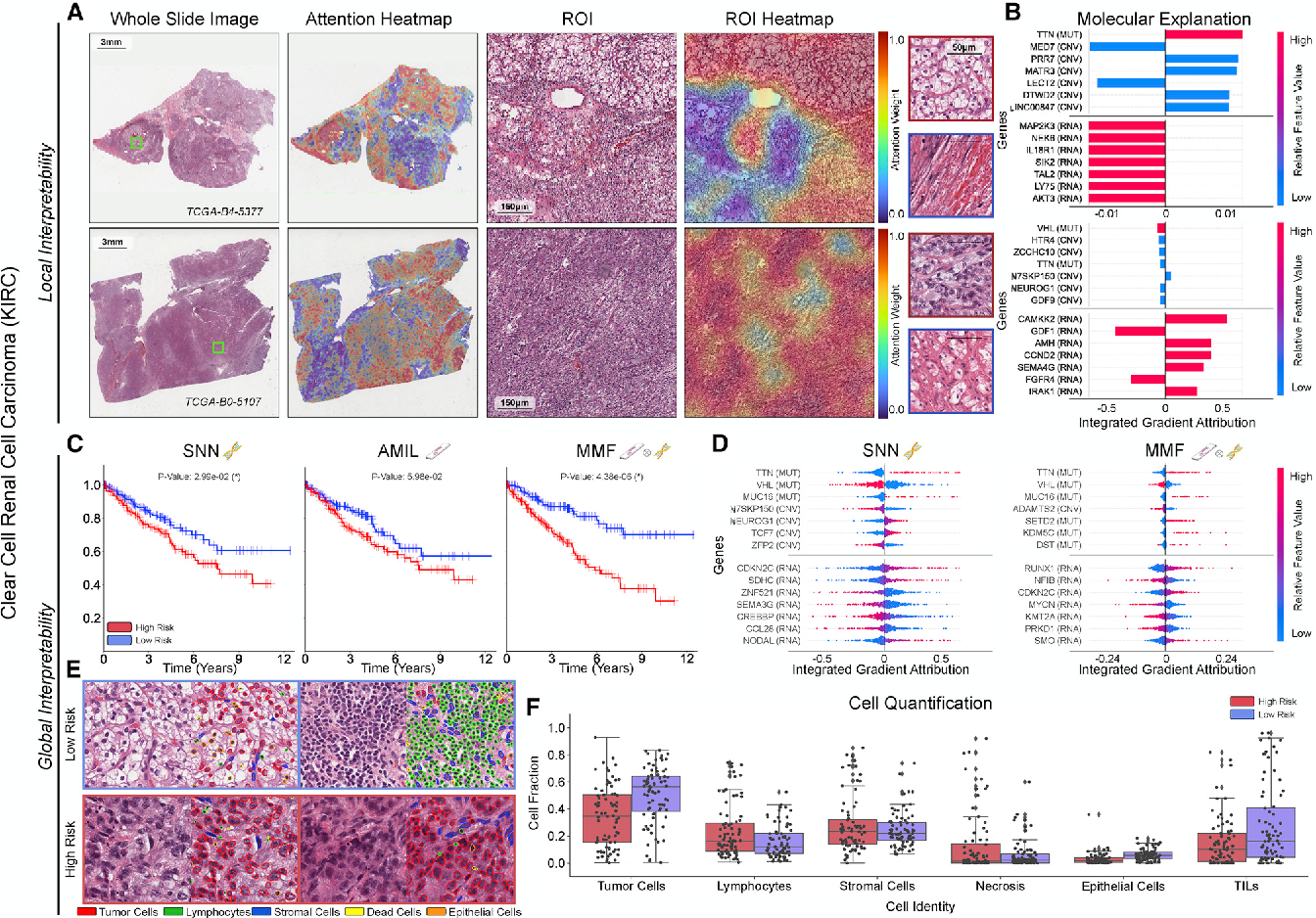
Figure 3 From Pan Cancer Integrative Histology Genomic Analysis Via We use multimodal deep learning to jointly examine pathology whole slide images and molecular profile data from 14 cancer types. our weakly supervised, multimodal deep learning algorithm is able to fuse these heterogeneous modalities to predict outcomes and discover prognostic features that correlate with poor and favorable outcomes. We use multimodal deep learning to jointly examine pathology whole slide images and molecular profile data from 14 cancer types. our weakly supervised, multimodal deep learning algorithm is able to fuse these heterogeneous modalities to predict outcomes and discover prognostic features that correlate with poor and favorable outcomes.
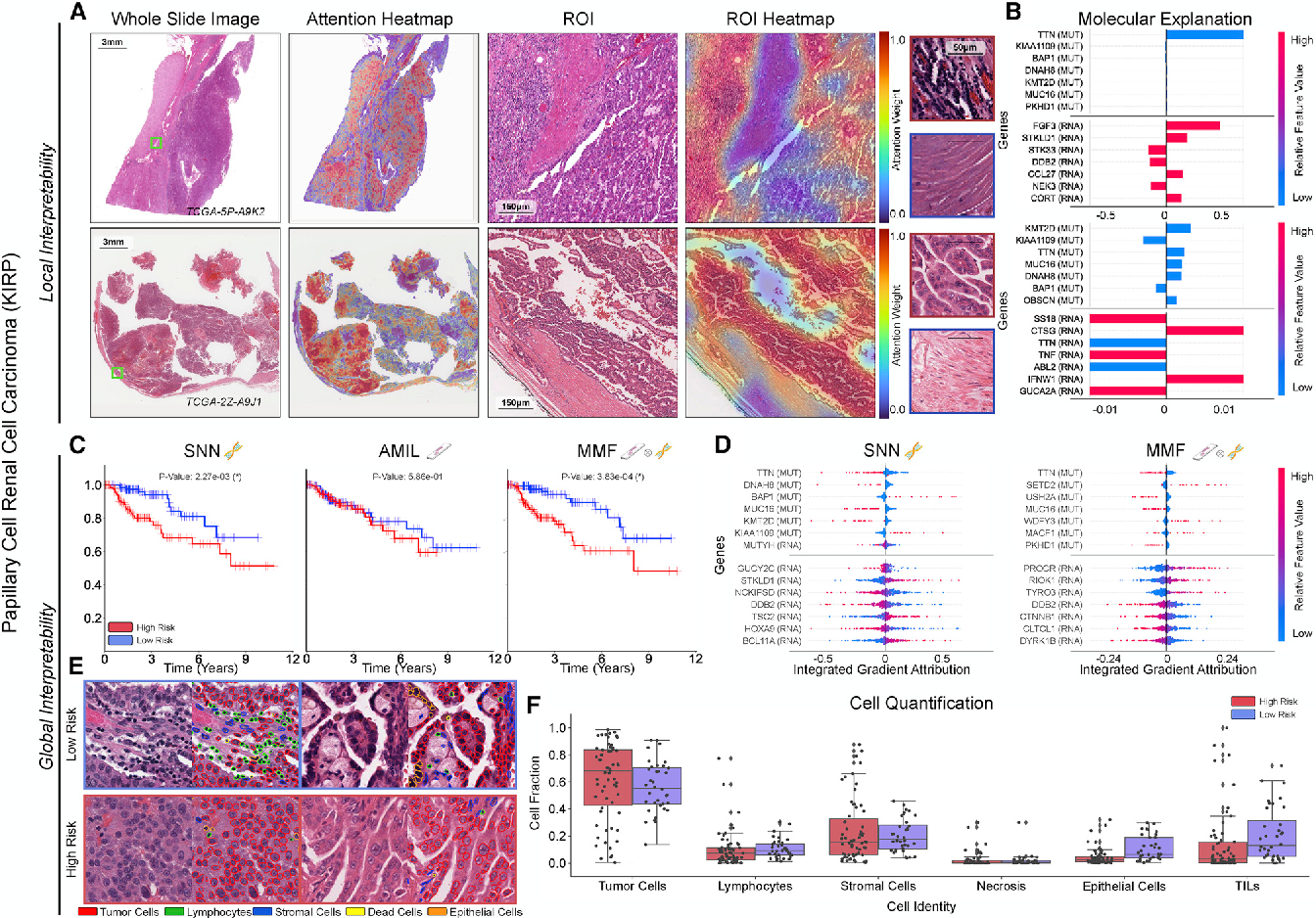
Figure 4 From Pan Cancer Integrative Histology Genomic Analysis Via We compared our model with unimodal deep learning models trained on histology slides and molecular profiles alone, and demonstrate performance increase in risk stratification on 9 out of 14 cancers. in addition, we analyze morphologic and molecular markers responsible for prognostic predictions across all cancer types. We use multimodal deep learning to jointly examine pathology whole slide images and molecular profile data from 14 cancer types. our weakly supervised, multimodal deep learning algorithm is able to fuse these heterogeneous modalities to predict outcomes and discover prognostic features that correlate with poor and favorable outcomes. We compared our model with unimodal deep learning models trained on histology slides and molecular profiles alone, and demonstrate performance increase in risk stratification on 9 out of 14 cancers. in addition, we analyze morphologic and molecular markers responsible for prognostic predictions across all cancer types. We present all analyses for morphological and molecular correlates of patient prognosis across the 14 cancer types at both a disease and a patient level in an interactive open access database to allow for further exploration, biomarker discovery, and feature assessment.
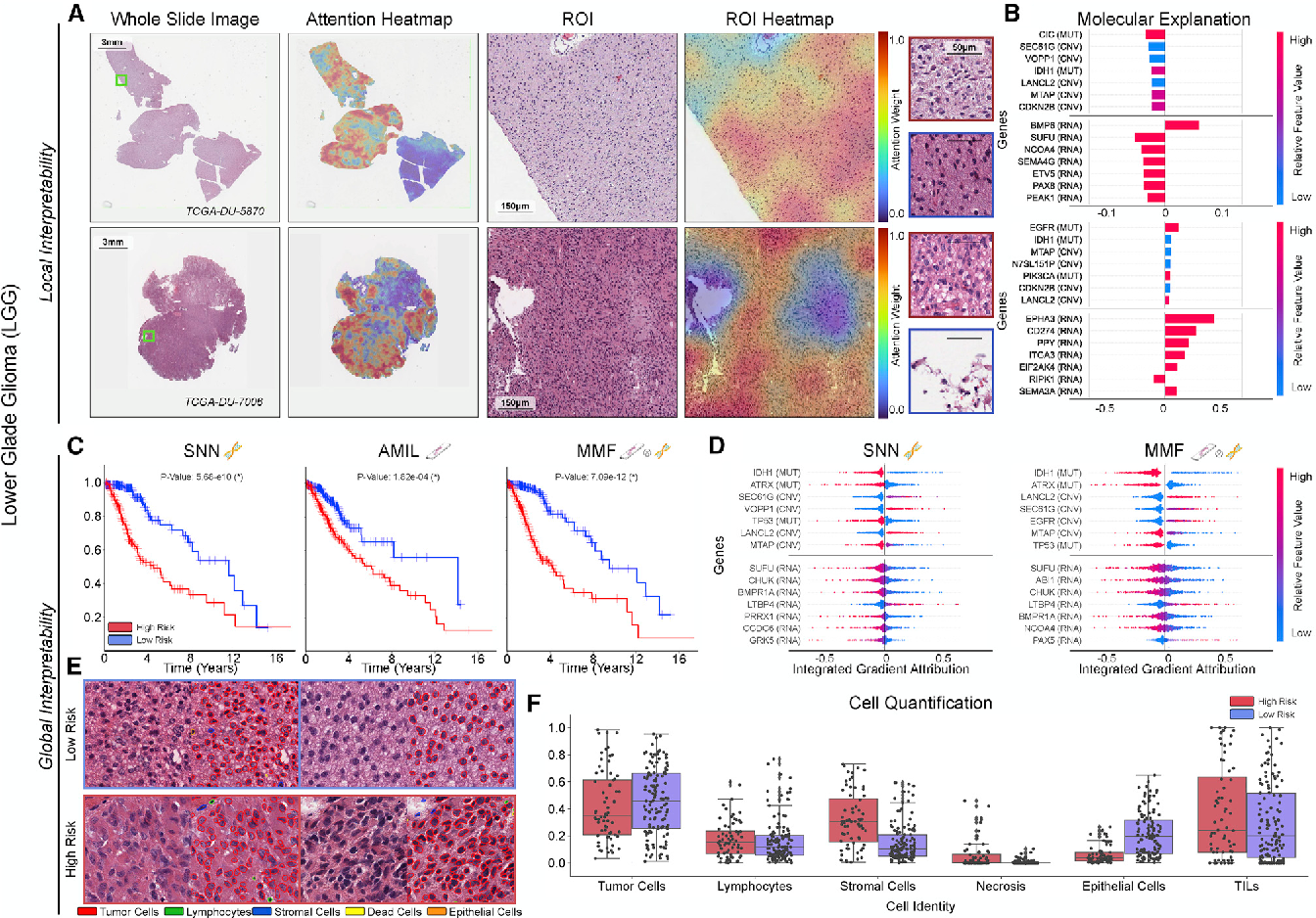
Figure 5 From Pan Cancer Integrative Histology Genomic Analysis Via We compared our model with unimodal deep learning models trained on histology slides and molecular profiles alone, and demonstrate performance increase in risk stratification on 9 out of 14 cancers. in addition, we analyze morphologic and molecular markers responsible for prognostic predictions across all cancer types. We present all analyses for morphological and molecular correlates of patient prognosis across the 14 cancer types at both a disease and a patient level in an interactive open access database to allow for further exploration, biomarker discovery, and feature assessment. We compared our model with unimodal deep learning models trained on histology slides and molecular profiles alone, and demonstrate performance increase in risk stratification on 9 out of 14 cancers. in addition, we analyze morphologic and molecular markers responsible for prognostic predictions across all cancer types. We used corresponding gigapixel whole slide images, rna seq abundance, copy number variation, and mutation data from 5,720 patients across 14 cancer types to train a weakly supervised, interpretable, multimodal deep learning algorithm that is able to not only fuse these heterogenous modalities for survival analysis, but also discover prognostic. We introduce a novel deep learning driven approach, catfusion, for pan cancer survival prediction by integrating multimodal histology genomic data. our methodology introduces several innovative elements. Background defensin beta 1 (defb1) is a key immune response gene, but its role in cancer remains unclear. this study aims to explore defb1 expression, genetic alterations, immune infiltration, and prognostic significance across various cancer types. methods we analyzed defb1 expression and its association with cancer prognosis using data from public platforms, including the cancer genome atlas.
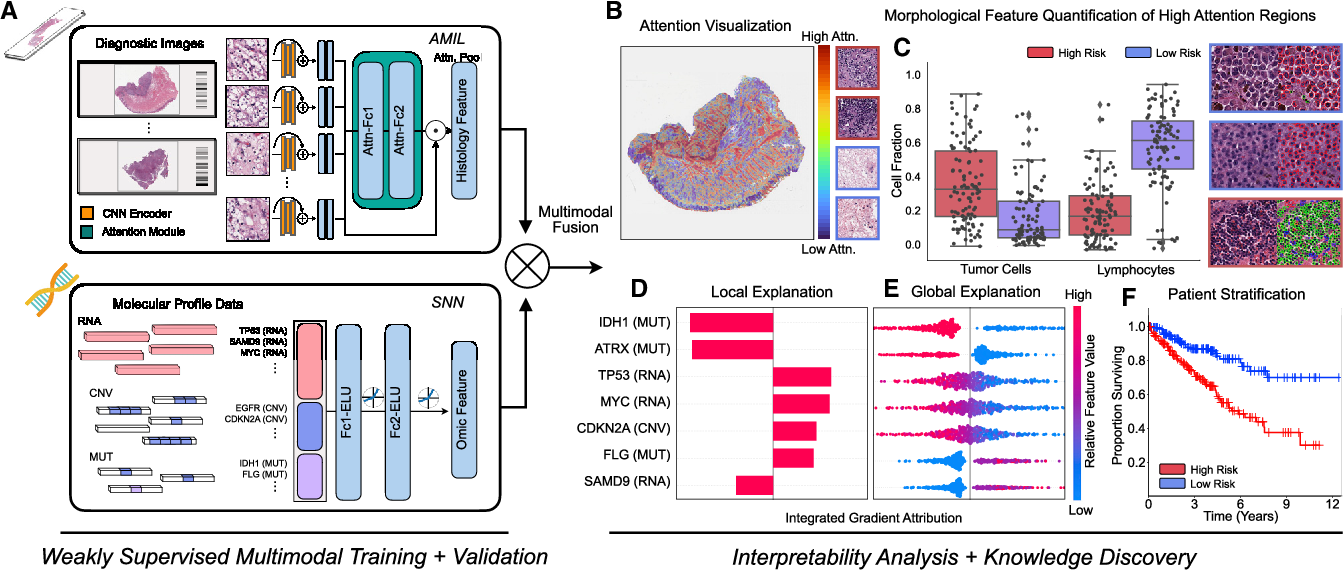
Figure 1 From Pan Cancer Integrative Histology Genomic Analysis Via We compared our model with unimodal deep learning models trained on histology slides and molecular profiles alone, and demonstrate performance increase in risk stratification on 9 out of 14 cancers. in addition, we analyze morphologic and molecular markers responsible for prognostic predictions across all cancer types. We used corresponding gigapixel whole slide images, rna seq abundance, copy number variation, and mutation data from 5,720 patients across 14 cancer types to train a weakly supervised, interpretable, multimodal deep learning algorithm that is able to not only fuse these heterogenous modalities for survival analysis, but also discover prognostic. We introduce a novel deep learning driven approach, catfusion, for pan cancer survival prediction by integrating multimodal histology genomic data. our methodology introduces several innovative elements. Background defensin beta 1 (defb1) is a key immune response gene, but its role in cancer remains unclear. this study aims to explore defb1 expression, genetic alterations, immune infiltration, and prognostic significance across various cancer types. methods we analyzed defb1 expression and its association with cancer prognosis using data from public platforms, including the cancer genome atlas.
Comments are closed.